QUITO-X: A New Perspective on Context Compression from the Information Bottleneck Theory
Aug 1, 2024·
,,,,,,,,·
0 min read

Yihang Wang
Xu Huang
Bowen Tian
Yueyang Su
Lei Yu
Humming Liao
Yixing Fan
Jiafeng Guo
Xueqi Cheng
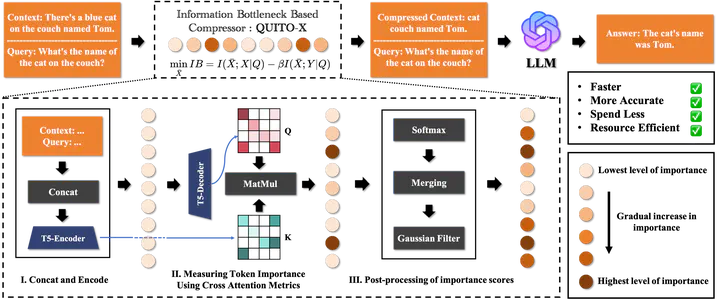
Abstract
Generative LLM have achieved remarkable success in various industrial applications, owing to their promising In-Context Learning capabilities. However, the issue of long context in complex tasks poses a significant barrier to their wider adoption, manifested in two main aspects: (i) The excessively long context leads to high costs and inference delays. (ii) A substantial amount of task-irrelevant information introduced by long contexts exacerbates the “lost in the middle” problem. Existing methods compress context by removing redundant tokens using metrics such as self-information or PPL, which is inconsistent with the objective of retaining the most important tokens when conditioning on a given query. In this study, we introduce information bottleneck theory (IB) to model the problem, offering a novel perspective that thoroughly addresses the essential properties required for context compression. Additionally, we propose a cross-attention-based approach to approximate mutual information in IB, which can be flexibly replaced with suitable alternatives in different scenarios. Extensive experiments on four datasets demonstrate that our method achieves a 25% increase in compression rate compared to the state-of-the-art, while maintaining question answering performance. In particular, the context compressed by our method even outperform the full context in some cases.
Type
Publication
arXiv preprint arXiv:2408.10497